Easily convert milliseconds to minutes and vice versa at Examples.com. Enter your data for quick and accurate results.
ms to min
Formula: Time in minutes(min) = Time in milliseconds(ms) ∕ 60000
Milliseconds :
Minutes :
milliseconds | minutes |
---|---|
1 | 0.000016666666666666667 |
min to ms
Formula: Time in milliseconds(ms) = Time in minutes(min)x 60000
Minutes :
Milliseconds :
minutes | milliseconds |
---|---|
1 | 60000 |
Time Converters to Millisecond (mm)
Time Converters to Minute (min)
Nanosecond to Minute | Microsecond to Minute | Millisecond to Minute |
Second to Minute | Hour to Minute | Day to Minute |
Week to Minute | Month to Minute | Calendar Year to Minute |
Decade to Minute | Century to Minute |
Conversion Factors:
- Milliseconds to Minutes: 1 minute = 60,000 milliseconds
- Minutes to Milliseconds: 1 millisecond = 1/60,000 minutes
How to Convert Milliseconds to Minutes:
To convert milliseconds to minutes, divide the number of milliseconds by 60,000.
Minutes=Milliseconds/60,000
Example:
Convert 120,000 milliseconds to minutes.
Minutes=120,000 ms/60,000=2 min
How to Convert Minutes to Milliseconds:
To convert minutes to milliseconds, multiply the number of minutes by 60,000.
Milliseconds=Minutes×60,000
Example:
Convert 3 minutes to milliseconds.
Milliseconds=3 min×60,000=180,000 ms
Milliseconds to Minutes Conversion Table
Milliseconds (ms) | Minutes (min) |
---|---|
1 ms | 1.66667e-5 min |
2 ms | 3.33333e-5 min |
3 ms | 5.00000e-5 min |
4 ms | 6.66667e-5 min |
5 ms | 8.33333e-5 min |
6 ms | 1.00000e-4 min |
7 ms | 1.16667e-4 min |
8 ms | 1.33333e-4 min |
9 ms | 1.50000e-4 min |
10 ms | 1.66667e-4 min |
20 ms | 3.33333e-4 min |
30 ms | 5.00000e-4 min |
40 ms | 6.66667e-4 min |
50 ms | 8.33333e-4 min |
60 ms | 1.00000e-3 min |
70 ms | 1.16667e-3 min |
80 ms | 1.33333e-3 min |
90 ms | 1.50000e-3 min |
100 ms | 1.66667e-3 min |
ms to min Conversion Chart
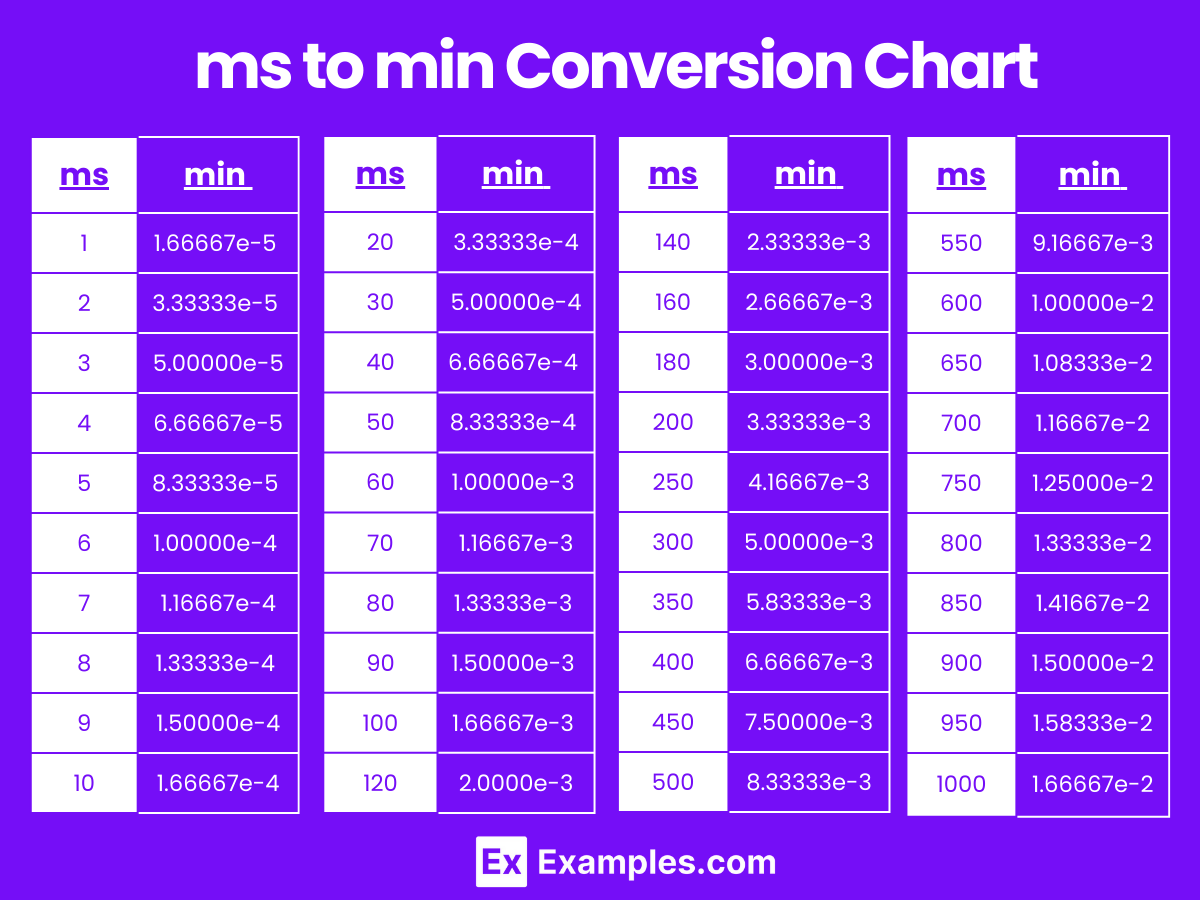
Minutes to Milliseconds Conversion Table
Minutes (min) | Milliseconds (ms) |
---|---|
1 min | 6.0e+4 ms |
2 min | 1.2e+5 ms |
3 min | 1.8e+5 ms |
4 min | 2.4e+5 ms |
5 min | 3.0e+5 ms |
6 min | 3.6e+5 ms |
7 min | 4.2e+5 ms |
8 min | 4.8e+5 ms |
9 min | 5.4e+5 ms |
10 min | 6.0e+5 ms |
20 min | 1.2e+6 ms |
30 min | 1.8e+6 ms |
40 min | 2.4e+6 ms |
50 min | 3.0e+6 ms |
60 min | 3.6e+6 ms |
70 min | 4.2e+6 ms |
80 min | 4.8e+6 ms |
90 min | 5.4e+6 ms |
100 min | 6.0e+6 ms |
min to ms Conversion Chart
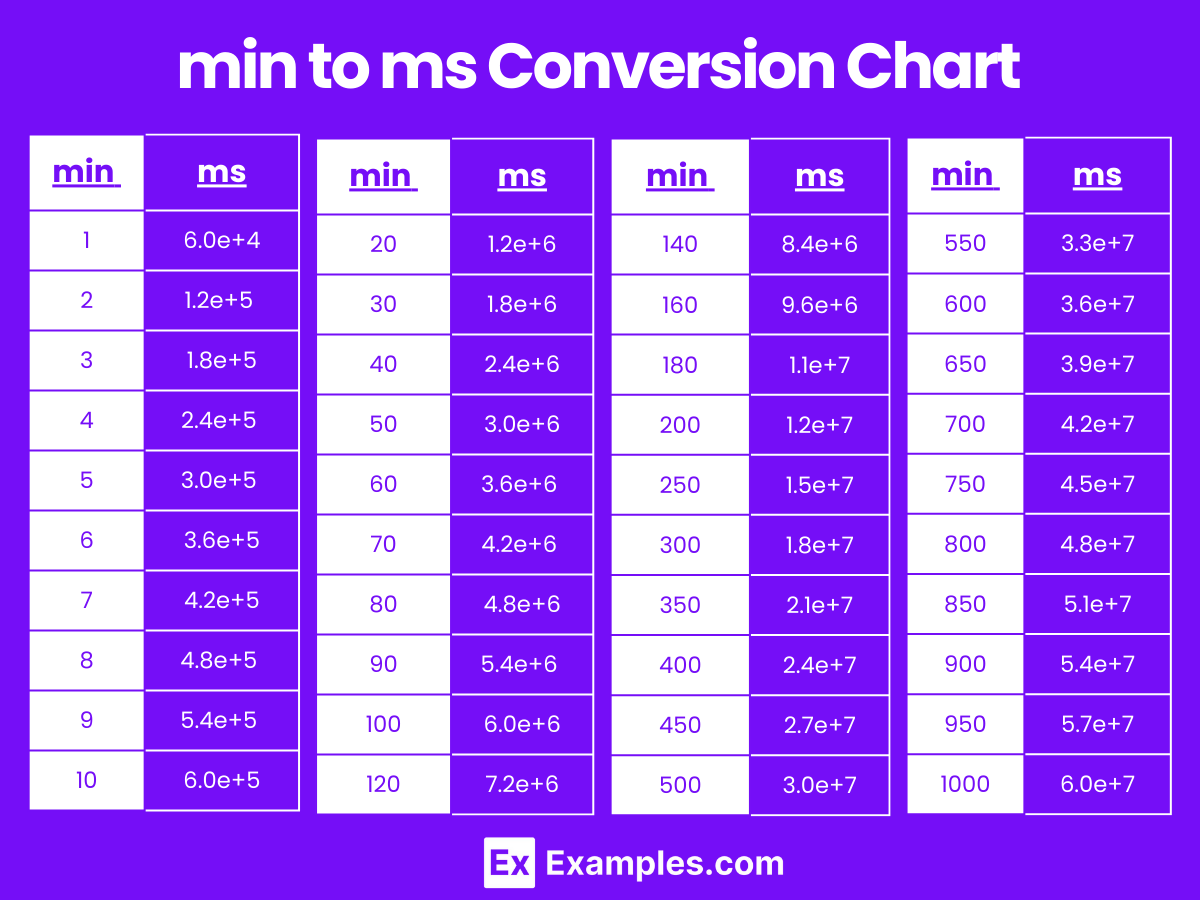
Difference Between Milliseconds to Minutes
Aspect | Milliseconds (ms) | Minutes (min) |
---|---|---|
Definition | A millisecond is one thousandth of a second. | A minute is equal to 60 seconds. |
Symbol | ms | min |
Conversion Factor | 1 ms = 0.00001667 min | 1 min = 60,000 ms |
Usage | Used for very precise time measurements. | Used for measuring longer time intervals. |
Precision | Suitable for high-precision applications like computing and scientific research. | Suitable for general time measurements in daily activities. |
Examples | 500 ms = 0.00833 min | 2 min = 120,000 ms |
Applications | Timing in sports, network latency, and performance metrics. | Scheduling, cooking, and standard timekeeping. |
Common Devices | Stopwatches, timers in software, and system clocks in computers. | Clocks, watches, timers, and scheduling devices. |
1. Solved Examples on Converting Milliseconds to Minutes
Example 1:
Convert 120,000 milliseconds to minutes.
Minutes=120,000 ms/60,000=2 min
Example 2:
Convert 300,000 milliseconds to minutes.
Minutes=300,000 ms/60,000=5 min
Example 3:
Convert 45,000 milliseconds to minutes.
Minutes=45,000 ms/60,000=0.75 min
Example 4:
Convert 600,000 milliseconds to minutes.
Minutes=600,000 ms/60,000=10 min
Example 5:
Convert 180,000 milliseconds to minutes.
Minutes=180,000 ms/60,000=3 min
2. Solved Examples on Converting Minutes to Milliseconds
Example 1:
Convert 2 minutes to milliseconds.
Milliseconds=2 min×60,000=120,000 ms
Example 2:
Convert 5 minutes to milliseconds.
Milliseconds=5 min×60,000=300,000 ms
Example 3:
Convert 0.75 minutes to milliseconds.
Milliseconds=0.75 min×60,000=45,000 ms
Example 4:
Convert 10 minutes to milliseconds.
Milliseconds=10 min×60,000=600,000 ms
Example 5:
Convert 3 minutes to milliseconds.
Milliseconds=3 min×60,000=180,000 ms
How do you quickly convert Milliseconds to Minutes?
To quickly convert milliseconds to minutes, you can move the decimal point four places to the left and multiply the result by 6. For example, 120,000 milliseconds becomes 12 multiplied by 0.6, which equals 2 minutes.
Why do we use 60,000 to convert Milliseconds to Minutes?
We use 60,000 to convert milliseconds to minutes because there are 60,000 milliseconds in one minute, as defined by the metric system.
How does converting Milliseconds to Minutes impact event scheduling?
Converting milliseconds to minutes helps in scheduling events by providing a more understandable time format, which is crucial for planning and coordinating activities.
How do Milliseconds to Minutes conversions help in scientific experiments?
Milliseconds to minutes conversions are important in scientific experiments for accurately recording and analyzing time intervals, especially when dealing with longer experimental durations.
Why is it important to convert Milliseconds to Minutes?
Converting milliseconds to minutes is important for understanding and managing time intervals in a more comprehensible and usable format, especially in applications that deal with longer durations.