15+ Cluster Sampling Examples to Download
Cluster sampling is a statistical sampling technique where the population is divided into separate groups, known as clusters. A random sample of these clusters is then selected, and all or a random sample of the individuals within the chosen clusters are included in the study. In contexts such as group communication, cluster sampling can help gather diverse perspectives from different segments of the population. When preparing a group project proposal or a group proposal, cluster sampling can ensure that the sample is representative of the broader group, enhancing the reliability and validity of the findings.
What is Cluster Sampling?
Cluster sampling is a method of sampling in which the entire population is divided into groups, or clusters, and a random sample of these clusters is selected. All members or a random selection of members from these chosen clusters are then included in the sample. This technique is especially useful when the population is large and spread out over a wide area, making it difficult and costly to conduct a simple random sample.
Examples of Cluster Sampling
- Selecting classrooms from a school to survey student opinions
- Choosing specific neighborhoods in a city to study household energy consumption
- Picking several departments within a company to assess employee satisfaction
- Randomly selecting a few branches of a bank to analyze customer service quality
- Choosing a number of rural villages to evaluate healthcare access
- Selecting specific hospitals in a region to study patient experiences
- Randomly picking a few stores in a retail chain to survey customer preferences
- Choosing certain university campuses to research student lifestyle habits
- Selecting a number of cities to study urban transportation patterns
- Picking specific farms within an agricultural area to study crop yields
- Choosing a few sports teams from a league to examine athlete performance
- Randomly selecting a number of government offices to assess public service efficiency
- Choosing certain factories in an industrial zone to evaluate worker safety
- Selecting a few libraries in a county to study reading habits
- Randomly picking several restaurants in a city to survey dining preferences
Types of Cluster Sampling
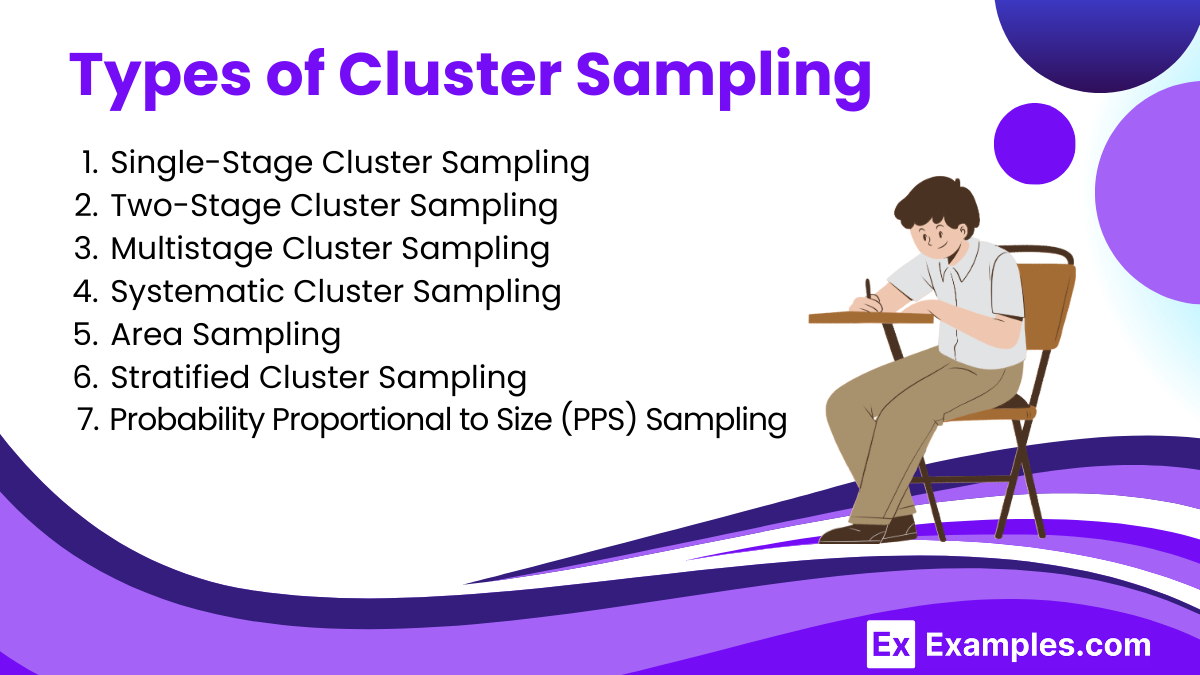
Cluster sampling is a method where the population is divided into groups, or clusters, and a random sample of these clusters is selected. There are several types of cluster sampling methods, each with its own approach:
1. Single-Stage Cluster Sampling
In single-stage cluster sampling, the researcher selects a random sample of clusters and includes all members of the chosen clusters in the sample.
2. Two-Stage Cluster Sampling
In two-stage cluster sampling, the researcher first selects a random sample of clusters. Then, within each selected cluster, a random sample of elements is chosen. This method reduces the overall sample size and cost.
3. Multistage Cluster Sampling
Multistage cluster sampling involves multiple levels of sampling. For example, in the first stage, clusters are selected; in the second stage, sub-clusters are chosen within each selected cluster; and so on. This process continues until the final sample of elements is selected.
4. Systematic Cluster Sampling
In systematic cluster sampling, clusters are selected using a systematic approach, such as selecting every nth cluster from a list. This method ensures an even spread of clusters across the population.
5. Area Sampling
Area sampling is a form of cluster sampling where clusters are defined geographically. This method is often used in studies that require spatial or regional analysis.
6. Stratified Cluster Sampling
In stratified cluster sampling, the population is first divided into strata (groups) based on a specific characteristic. Then, clusters are randomly selected from each stratum. This method combines the benefits of stratified sampling and cluster sampling.
This technique is particularly useful when studying ethnic groups within a population, ensuring that each group is adequately represented. Additionally, when evaluating group communication skills, stratified cluster sampling allows researchers to gather data from diverse groups, providing a comprehensive understanding of communication dynamics across different strata.
7. Probability Proportional to Size (PPS) Sampling
In PPS sampling, clusters are selected based on their size relative to the total population. Larger clusters have a higher probability of being selected, ensuring that the sample is representative of the population distribution.
Steps to Conduct Cluster Sampling
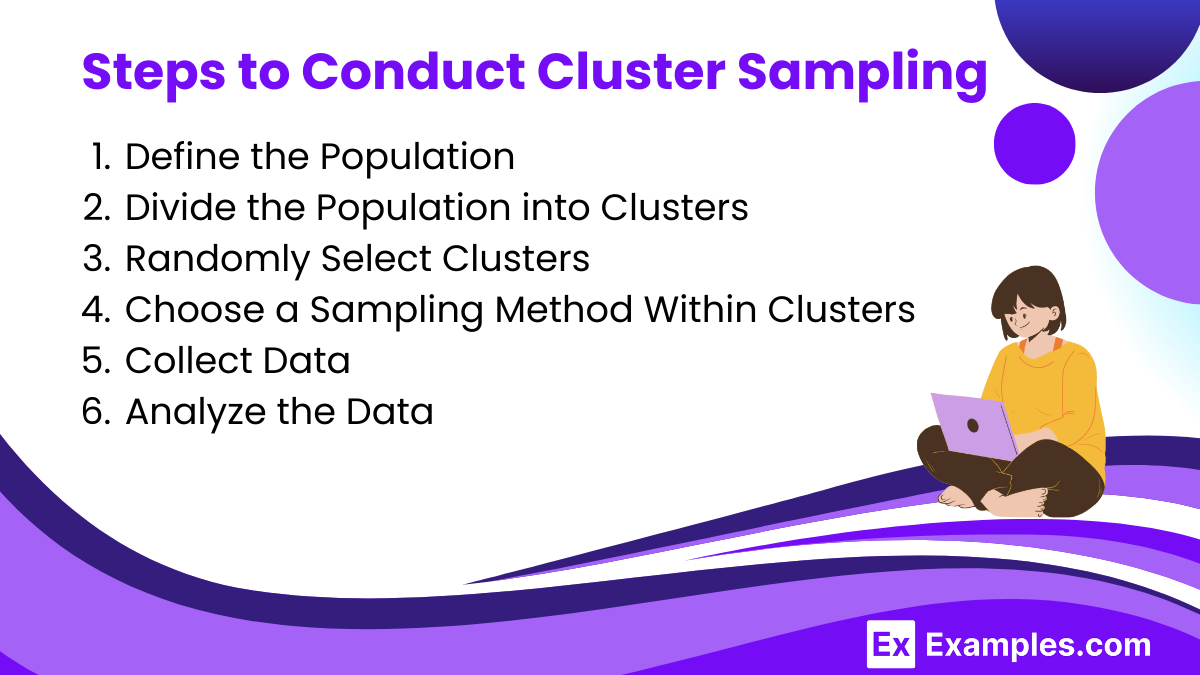
1. Define the Population
Identify the entire population from which you want to draw a sample.
2. Divide the Population into Clusters
Group the population into clusters, ensuring that each cluster is mutually exclusive and collectively exhaustive.
3. Randomly Select Clusters
Use a random sampling method to select a certain number of clusters from the population.
4. Choose a Sampling Method Within Clusters
Decide whether to include all elements within the selected clusters (single-stage) or to sample elements within each cluster (two-stage or multistage).
5. Collect Data
Gather data from the selected clusters or sampled elements within those clusters.
6. Analyze the Data
Analyze the collected data to make inferences about the entire population.
Cluster Sampling in Statistics
Cluster sampling is a statistical method used to simplify the process of data collection by dividing the population into separate groups, known as clusters. Instead of sampling individuals from the entire population, researchers randomly select entire clusters and then collect data from every member within those selected clusters.
This technique can be particularly effective when comparing interpersonal communication vs group communication, as it allows researchers to gather data from distinct groups efficiently. In a statistics report, using cluster sampling can help provide clear insights and representative data. Additionally, this method can be beneficial when writing an essay on population, as it enables the study of population characteristics without the need for a complete census.
Steps in Cluster Sampling
- Identify Clusters: Define the clusters within the population. Clusters should be mutually exclusive and collectively exhaustive.
- Randomly Select Clusters: Use a random sampling method to choose a set of clusters.
- Collect Data: Gather data from every member within the selected clusters.
Cluster Sampling Advantages & Disadvantages
Advantages | Disadvantages |
---|---|
Cost-Effective | Less Precision |
Reduces travel and administrative costs by concentrating data collection within selected clusters. | Can lead to higher sampling error if clusters are not well-defined or vary greatly in size. |
Feasible for Large Populations | Homogeneity within Clusters |
Practical for large or dispersed populations where other sampling methods would be impractical. | If clusters are too homogeneous, the sample may not adequately represent the population. |
Simplicity | Complex Analysis |
Simplifies the sampling process, especially when a complete list of the population is not available. | Data analysis can be more complex due to the multi-stage nature of the sampling. |
Reduced Time and Effort | Cluster Size Variability |
Requires less time and effort compared to sampling individuals from a dispersed population. | Variability in cluster sizes can affect the representativeness and accuracy of the sample. |
Ease of Implementation | Potential Bias |
Easier to implement when population groups are naturally divided into clusters. | If clusters are not randomly selected, there is a risk of selection bias. |
Cluster Sampling vs Stratified Sampling
Aspect | Cluster Sampling | Stratified Sampling |
---|---|---|
Definition | Divides the population into clusters and randomly selects entire clusters. | Divides the population into strata and randomly samples from each stratum. |
Population Division | Based on naturally occurring groups or clusters. | Based on specific characteristics or strata such as age, gender, income. |
Sampling Process | Randomly selects clusters, then samples all individuals within those clusters. | Randomly selects individuals from each stratum. |
Homogeneity | Clusters are heterogeneous within but homogeneous between clusters. | Strata are homogeneous within but heterogeneous between strata. |
Ease of Implementation | Easier to implement when clusters are naturally occurring. | Requires detailed knowledge of population characteristics to create strata. |
Cost | Generally more cost-effective for large, dispersed populations. | Can be more costly and time-consuming due to detailed stratification. |
Precision | Less precision due to potential intra-cluster homogeneity. | Higher precision due to reduced sampling error within each stratum. |
Example Use Case | Surveying households in different neighborhoods. | Surveying different age groups within a population. |
Data Collection | Collects data from all individuals within selected clusters. | Collects data from a sample of individuals from each stratum. |
Bias Risk | Risk of higher sampling error if clusters are not well-defined. | Lower risk of sampling error, provided strata are well-defined and proportional. |
Analysis Complexity | Can involve more complex data analysis due to multi-stage sampling. | Simplifies analysis as each stratum can be analyzed separately and then combined. |
Applications of Cluster Sampling
- Sociological Research: Sociologists use cluster sampling to investigate social behaviors and attitudes. For instance, they might divide a population into clusters based on demographic factors such as age, income, or ethnicity, and then select clusters to study the social dynamics or cultural practices within those groups.
- Political Polling: In political polling, cluster sampling helps to gauge public opinion or voting intentions. Pollsters might divide a state into electoral districts (clusters) and randomly select districts to survey all eligible voters about their political preferences and opinions on various issues.
- Healthcare Surveys: Healthcare providers and policymakers use cluster sampling to evaluate healthcare access and quality. They might divide a region into healthcare service areas (clusters) and select a few areas to survey all residents about their healthcare experiences and needs.
- Agricultural Research: Cluster sampling is applied in agricultural studies to assess crop yields, pest infestations, or farming practices. Researchers might divide farmland into plots (clusters) and randomly select plots to study all crops or farming activities within those plots.
- Epidemiological Studies: In epidemiology, cluster sampling helps to investigate the spread of diseases and risk factors. Researchers might divide a city into zones (clusters) and select zones to study the health status and risk behaviors of all residents within those zones.
- Government Surveys: Government agencies use cluster sampling for large-scale surveys such as the census or labor force surveys. They might divide a country into administrative areas (clusters) and randomly select areas to collect data from all households or individuals within those areas.
How do you perform cluster sampling?
Divide the population into clusters, randomly select clusters, and then collect data from all members of chosen clusters.
What are clusters in sampling?
Clusters are naturally occurring, homogeneous groups within a population, like neighborhoods or schools.
What is a key advantage of cluster sampling?
Cluster sampling is cost-effective and practical for large, geographically spread populations.
What is a disadvantage of cluster sampling?
The main disadvantage is the potential for increased sampling error compared to other methods.
How does cluster sampling differ from stratified sampling?
In stratified sampling, researchers select individuals from each stratum, while in cluster sampling, they select entire clusters.
Can cluster sampling be used for any population?
Cluster sampling works best for populations naturally divided into homogeneous clusters.
What is a two-stage cluster sampling?
Two-stage cluster sampling involves selecting clusters first and then sampling individuals within those clusters.
When is cluster sampling most effective?
Cluster sampling is most effective when clusters are similar to each other in key characteristics.
What is the difference between single-stage and multi-stage cluster sampling?
Single-stage involves sampling all individuals in selected clusters, while multi-stage samples individuals within selected clusters.
How does cluster sampling improve efficiency?
Cluster sampling reduces travel and administrative costs by focusing on specific, manageable clusters.