15+ Stratified Sampling Examples to Download
Stratified sampling is a statistical method of sampling that involves dividing a population into distinct subgroups or strata that share similar characteristics, and then randomly selecting samples from each stratum. In contrast to stratified sampling, photography cluster sampling might involve selecting clusters of events or locations to photograph, and then capturing images from within those clusters. Simple random sampling randomly selects individuals from the entire population without considering subgroups, while quota sampling involves selecting samples that meet specific criteria until a pre-set quota is reached, ensuring representation of certain characteristics within the population.
What is Stratified Sampling?
Stratified sampling is a method used in statistics where a population is divided into smaller groups, or strata, based on shared characteristics. Samples are then randomly selected from each of these groups. In research studies, maintaining photography internal external validity is crucial to ensure the findings are credible and applicable in different contexts. This method can be beneficial in brand marketing to ensure diverse consumer segments are accurately represented in the analysis. Additionally, incorporating stratified sampling into a data gathering procedure can enhance the precision and reliability of the collected data, ensuring comprehensive coverage of the populationās subgroups.
Examples of Stratified Sampling
- Sampling students from different grade levels in a school
- Surveying employees from various departments in a company
- Selecting patients from different age groups in a hospital
- Choosing voters from different political affiliations in an election study
- Surveying households from different income brackets in a city
- Selecting participants from different ethnic groups in a cultural study
- Sampling plants from different species in a botanical garden
- Choosing respondents from different geographic regions in a national survey
- Selecting athletes from different sports teams for a fitness study
- Surveying customers from different membership levels in a retail store
- Sampling residents from different neighborhoods in a city
- Selecting books from different genres in a library study
- Surveying users from different age groups on a social media platform
- Choosing patients from different medical conditions in a health study
- Sampling businesses from different industries in an economic survey
Types of Stratified Sampling
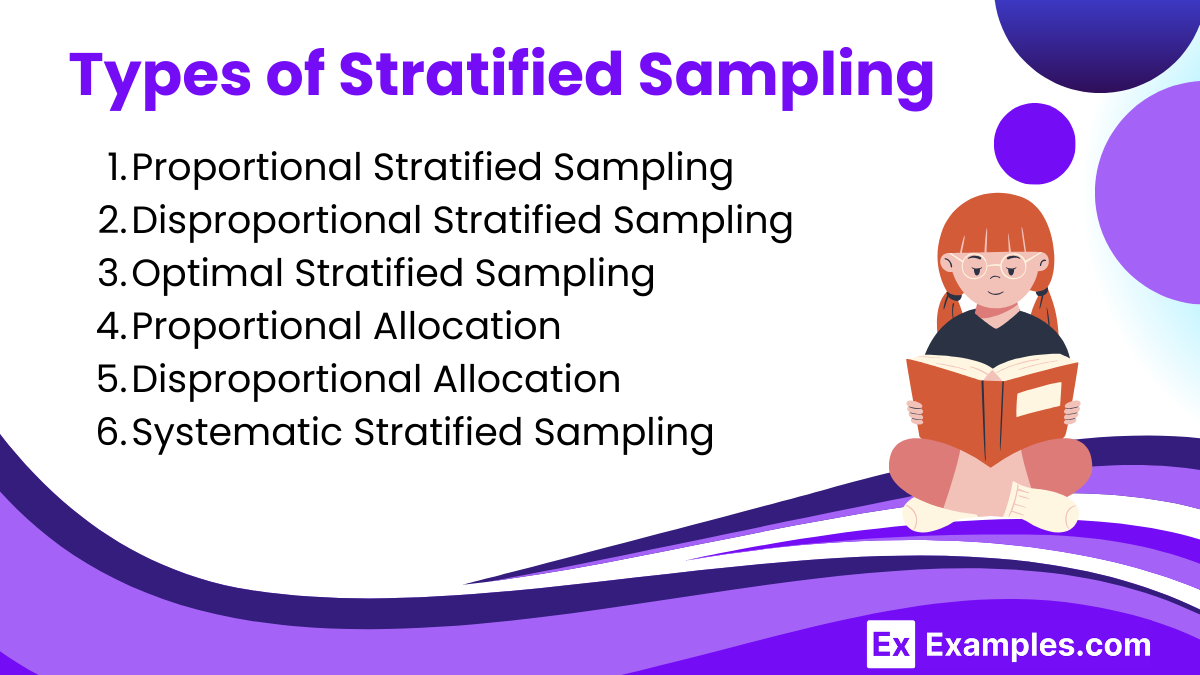
1. Proportional Stratified Sampling
- Description: The sample size from each stratum is proportional to the stratumās size in the population.
- Example: If a school has 60% juniors and 40% seniors, a proportional stratified sample would include 60% juniors and 40% seniors in the sample.
2. Disproportional Stratified Sampling
- Description: The sample size from each stratum is not proportional to the stratumās size in the population. This approach is used when certain strata are more important or variable, and thus require a larger sample size.
- Example: In a company where the management team is small but critical, you might sample 50 managers out of 200, even if they make up only 5% of the population.
3. Optimal Stratified Sampling
- Description: A variation of disproportional sampling where the sample size from each stratum is determined based on both the size and variability of the stratum, aiming to minimize variance and cost.
- Example: More samples are taken from strata with higher variability and fewer from those with lower variability, regardless of the stratumās population size.
4. Proportional Allocation
- Description: The overall sample size is divided among the strata in proportion to the size of each stratum.
- Example: If you need a total sample size of 100 and the population has 30% men and 70% women, you would sample 30 men and 70 women.
5. Disproportional Allocation
- Description: Samples are allocated to strata not in proportion to their sizes but based on other criteria, such as the importance of the stratum or expected variance.
- Example: Allocating more resources to sample a critical but small subgroup heavily impacted by the research subject.
6. Systematic Stratified Sampling
- Description: Combines systematic sampling and stratified sampling. After dividing the population into strata, a systematic sampling method (e.g., every 10th item) is used within each stratum.
- Example: In a population of 1000, you divide it into 5 strata and systematically sample every 5th individual within each stratum.
When to use Stratified Sampling
- Heterogeneous Populations: When the population is diverse and contains distinct subgroups that are expected to have different characteristics. Stratified sampling ensures that each subgroup is adequately represented.
- Ensuring Representation: When it is crucial to ensure that specific subgroups within the population are represented in the sample. This is important for studies where the characteristics of these subgroups are of particular interest.
- Reducing Variability: To reduce sampling error and increase the precision of the estimates. By ensuring each subgroup is proportionately represented, stratified sampling can provide more accurate results than simple random sampling.
- Small Subgroups: When some subgroups are small but significant, and there is a risk they might be underrepresented or missed entirely in a random sample. Stratified sampling guarantees their inclusion.
- Comparative Studies: When comparing specific subgroups within the population. For instance, in market research, comparing the preferences of different age groups, income levels, or geographic regions.
- Resource Allocation: When resources are limited, and you want to ensure that the sample accurately reflects the populationās diversity without over-sampling large subgroups.
Simple vs. Stratified Samples
Aspect | Simple Random Sampling | Stratified Sampling |
---|---|---|
Definition | Every member has an equal chance of selection. | Population divided into subgroups, random samples taken from each. |
Population Assumption | Homogeneous or similar characteristics. | Heterogeneous with distinct subgroups. |
Implementation | Simple and straightforward. | More complex, requires identifying strata. |
Accuracy | May not adequately represent all subgroups. | Ensures proportional representation of all subgroups. |
Sampling Error | Potentially higher if population is diverse. | Lower due to representation of all relevant subgroups. |
Example | Randomly selecting employees from a company. | Selecting employees based on department and then randomly within each department. |
Cluster Sampling Vs. Stratified Sampling
Aspect | Cluster Sampling | Stratified Sampling |
---|---|---|
Definition | Population divided into clusters, entire clusters sampled. | Population divided into strata, random samples from each stratum. |
Division Criteria | Naturally occurring clusters (e.g., geographic, organizational). | Specific characteristics (e.g., age, gender, income). |
Selection Process | Randomly select entire clusters, sample all members within them. | Randomly select individuals from each stratum. |
Homogeneity Assumption | Within clusters. | Within strata. |
Cost and Logistics | More cost-effective, easier for large, dispersed populations. | More complex, potentially more expensive. |
Precision | Less precise if clusters vary greatly. | More precise due to representation of all subgroups. |
Sampling Error | Higher potential if clusters are not representative. | Lower due to proportional representation of subgroups. |
Example | Sampling all employees from randomly selected company branches. | Sampling employees from each department based on department size. |
Stratified Sampling Vs. Quota Sampling
Aspect | Stratified Sampling | Quota Sampling |
---|---|---|
Definition | Population divided into strata, random samples from each stratum. | Population divided into subgroups, non-random samples until quota met. |
Division Criteria | Specific characteristics (e.g., age, gender, income). | Specific characteristics (e.g., age, gender, income). |
Selection Process | Random sampling within each stratum. | Non-random sampling until quotas are filled. |
Homogeneity Assumption | Within strata. | Within subgroups. |
Representation | Proportional representation of all subgroups. | Ensures representation but not necessarily proportional. |
Precision | Higher precision, lower sampling error. | Lower precision, higher potential for bias. |
Sampling Error | Reduced due to proportional representation. | Increased due to non-random selection. |
Complexity and Time | More complex and time-consuming. | Easier and quicker to implement. |
Example | Sampling from age groups proportionally. | Sampling a fixed number from each gender group non-randomly. |
Advantages and Disadvantages of Stratified Sampling
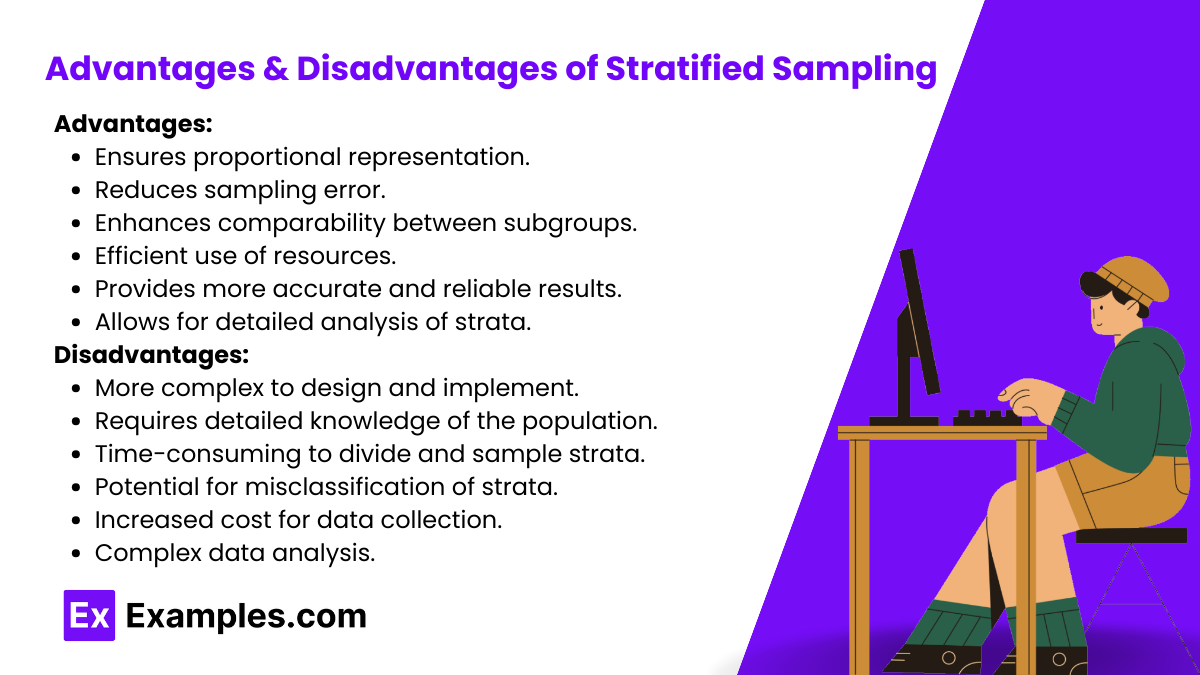
- Advantages:
- Ensures proportional representation.
- Reduces sampling error.
- Enhances comparability between subgroups.
- Efficient use of resources.
- Provides more accurate and reliable results.
- Allows for detailed analysis of strata.
- Disadvantages:
- More complex to design and implement.
- Requires detailed knowledge of the population.
- Time-consuming to divide and sample strata.
- Potential for misclassification of strata.
- Increased cost for data collection.
- Complex data analysis.
How do you create strata in stratified sampling?
Create strata based on characteristics relevant to the study, such as age, gender, income level, or education.
What are the types of stratified sampling?
The main types are proportional stratified sampling, where sample size in each stratum is proportional to the population, and equal stratified sampling, where each stratum has the same sample size.
When should you use stratified sampling?
Use it when the population has distinct subgroups, and you want to ensure each subgroup is represented in your sample.
How do you determine sample size in stratified sampling?
Determine sample size based on the desired precision, population size, and variability within and between strata.
What is proportional stratified sampling?
Proportional stratified sampling involves selecting a sample from each stratum proportional to its size in the population.
What is equal stratified sampling?
Equal stratified sampling involves selecting the same number of samples from each stratum, regardless of the stratum size.
How does stratified sampling reduce sampling error?
Stratified sampling reduces sampling error by ensuring each subgroup is represented, which decreases variability within the sample.
Can stratified sampling be used with other sampling methods?
Yes, you can combine stratified sampling with methods like random or systematic sampling for more refined results.
How do you ensure randomness in stratified sampling?
Ensure randomness by using random sampling techniques within each stratum after dividing the population.
What is the main goal of stratified sampling?
The main goal is to ensure all subgroups within a population are adequately represented in the sample.