Model Misspecification
- Notes
Understanding model misspecification is crucial for CFA Level 2 candidates, as it pertains to the integrity and accuracy of financial models used in quantitative analysis. Recognizing and correcting model misspecification helps ensure that financial models provide reliable insights for investment decisions, a key competency in the Quantitative Methods section of the exam.
Learning Objective
In studying “Model Misspecification” for the CFA exam, you should aim to master the identification and correction of misspecification errors in statistical models. This includes understanding the consequences of omitting variables, including irrelevant variables, or incorrectly assuming linearity or normality. Learning to detect these errors through diagnostic tests and adjusting models accordingly will enable you to enhance the robustness and validity of financial predictions and valuations.
1. Types of Model Misspecification
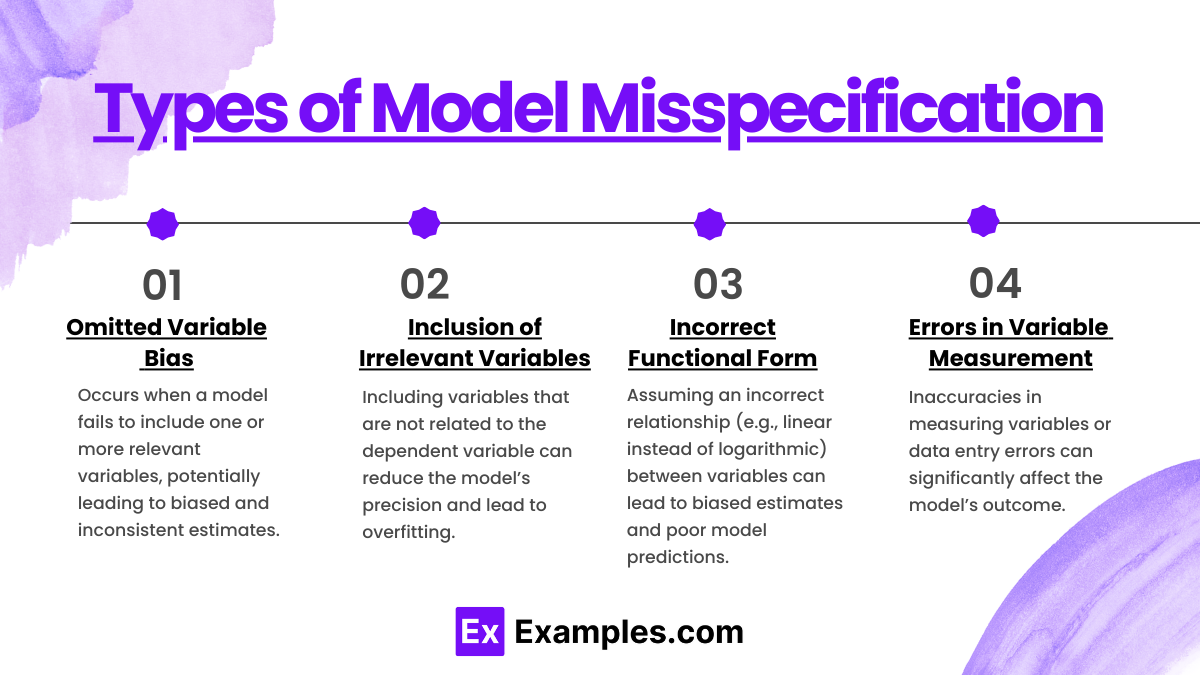
- Omitted Variable Bias: Occurs when a model fails to include one or more relevant variables, potentially leading to biased and inconsistent estimates.
- Inclusion of Irrelevant Variables: Including variables that are not related to the dependent variable can reduce the model’s precision and lead to overfitting.
- Incorrect Functional Form: Assuming an incorrect relationship (e.g., linear instead of logarithmic) between variables can lead to biased estimates and poor model predictions.
- Errors in Variable Measurement: Inaccuracies in measuring variables or data entry errors can significantly affect the model’s outcome.
2. Diagnosing Misspecification
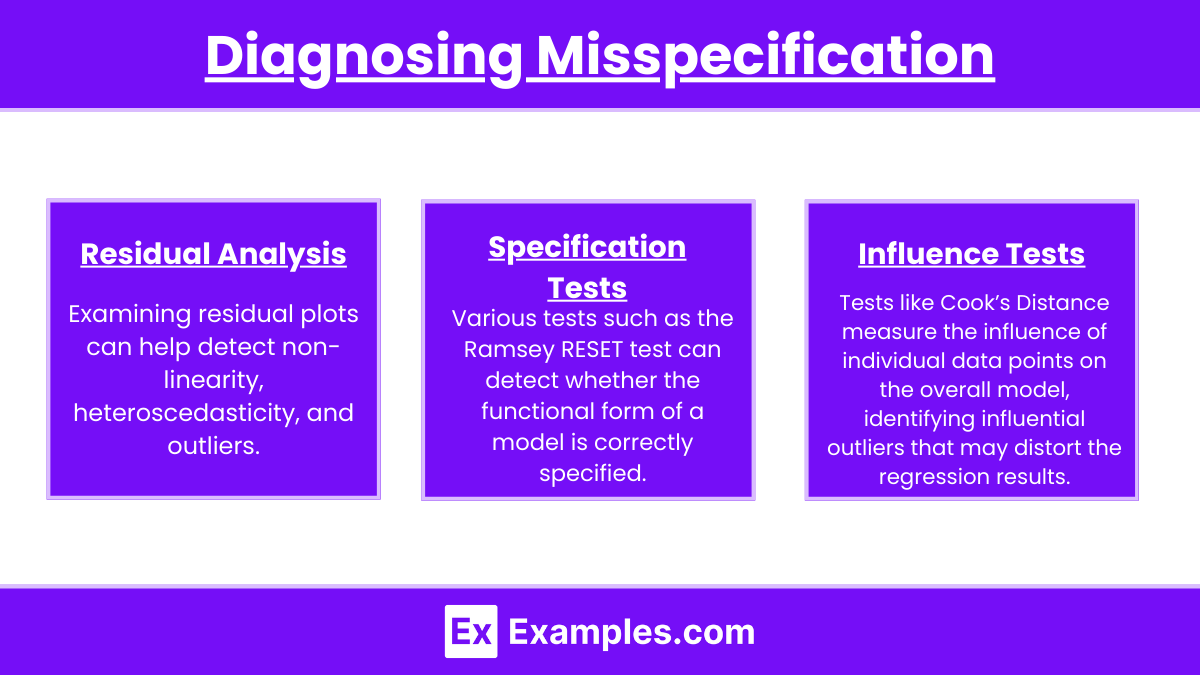
- Residual Analysis: Examining residual plots can help detect non-linearity, heteroscedasticity, and outliers.
- Specification Tests: Various tests such as the Ramsey RESET test can detect whether the functional form of a model is correctly specified.
- Influence Tests: Tests like Cook’s Distance measure the influence of individual data points on the overall model, identifying influential outliers that may distort the regression results.
3. Consequences of Model Misspecification
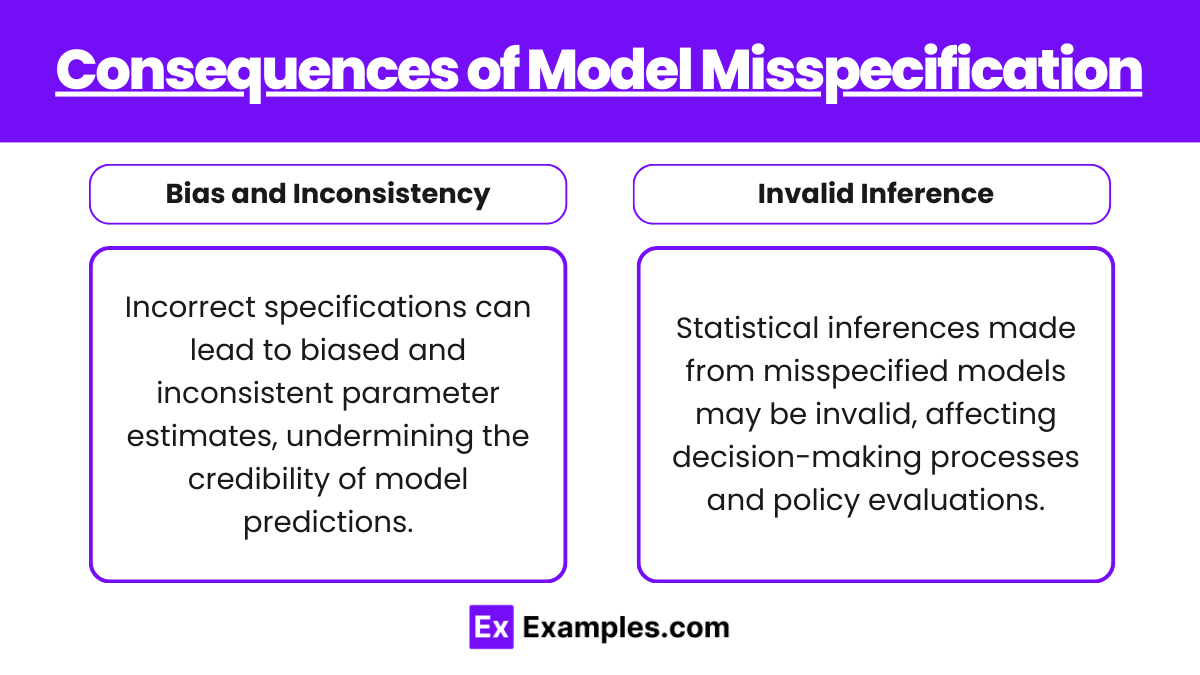
- Bias and Inconsistency: Incorrect specifications can lead to biased and inconsistent parameter estimates, undermining the credibility of model predictions.
- Invalid Inference: Statistical inferences made from misspecified models may be invalid, affecting decision-making processes and policy evaluations.
4. Correcting Misspecification
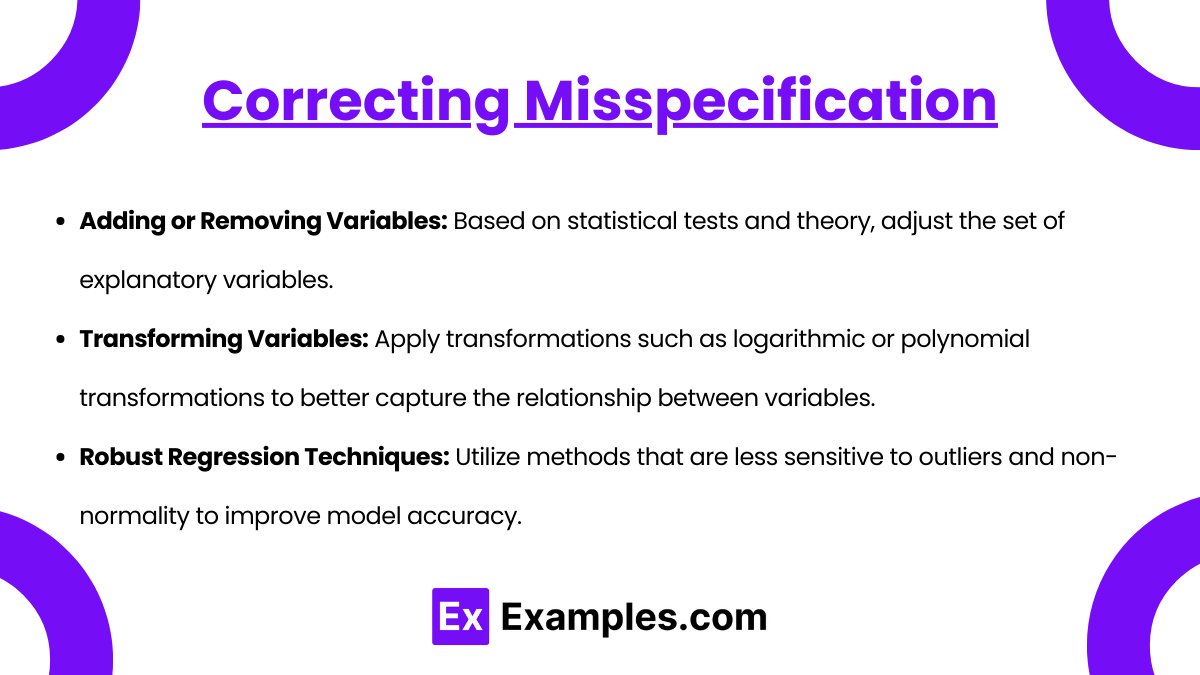
- Adding or Removing Variables: Based on statistical tests and theory, adjust the set of explanatory variables.
- Transforming Variables: Apply transformations such as logarithmic or polynomial transformations to better capture the relationship between variables.
- Robust Regression Techniques: Utilize methods that are less sensitive to outliers and non-normality to improve model accuracy.
Examples
Example 1: Omitted Variable Bias in Investment Analysis
- An analyst models stock returns without considering market volatility, resulting in underestimated risk premiums and potentially misguided investment strategies.
Example 2: Including Irrelevant Variables in Economic Modeling
- A model predicting consumer spending includes unrelated variables such as geographical latitude, diluting the model’s effectiveness and precision.
Example 3: Incorrect Functional Form in Real Estate Pricing
- A linear model is used to predict house prices based on square footage, ignoring the non-linear effects of location and property age, which skews valuation results.
Example 4: Measurement Error in Financial Data
- Errors in recording asset values due to miskeying data lead to incorrect estimations of asset depreciation rates in a corporate financial model.
Example 5: Influence of Outliers on Portfolio Performance
- An extreme stock return during a market anomaly significantly influences the average return calculation, misleading the portfolio performance assessment.
Practice Questions
Question 1:
What is a likely result of omitting a relevant variable from a regression model?
A) Reduced complexity of the model
B) Increased accuracy of the estimates
C) Biased regression coefficients
D) Higher R-squared values
Answer: C) Biased regression coefficients
Explanation: Omitting an important variable from a regression model typically leads to biased and inconsistent estimates of the regression coefficients, as the omitted variable’s effect is wrongly attributed to other variables.
Question 2:
Which test is used to check for correct functional form in a regression model?
A) Durbin-Watson test
B) Ramsey RESET test
C) F-test
D) AIC criterion
Answer: B) Ramsey RESET test
Explanation: The Ramsey RESET test is specifically designed to assess whether the functional form of a model is correctly specified, helping detect whether additional non-linear transformations of the predictors might be necessary.
Question 3:
How can the influence of outliers be mitigated in a regression analysis?
A) By increasing the sample size
B) Through the use of robust regression methods
C) By removing all outliers
D) By adding more predictors
Answer: B) Through the use of robust regression methods
Explanation: Robust regression methods are designed to lessen the impact of outliers and leverage points in the data, thereby improving the reliability and stability of the model estimates without necessarily removing all outliers.