Preparing for the CFA Exam requires proficiency in “Simulation Methods,” including Monte Carlo simulations and scenario analysis, to model uncertainty, assess risks, and forecast outcomes in complex financial scenarios. These skills are essential for informed, data-driven decisions in portfolio management and valuation, helping candidates succeed on the CFA Exam.
Learning Objectives
In studying “Simulation Methods” for the CFA exam, you should learn to understand the role of simulation techniques in financial risk analysis and decision-making. These methods enable analysts to model complex financial scenarios by generating a range of possible outcomes, incorporating the uncertainties of real-world variables. Learn about common simulation applications like Monte Carlo simulations, scenario analysis, and risk modeling for portfolios. Recognize how simulations can reveal potential risk exposures and help in stress-testing financial models, providing insights into the probability of various outcomes that inform sound investment and risk management decisions.
Types of Simulation Methods
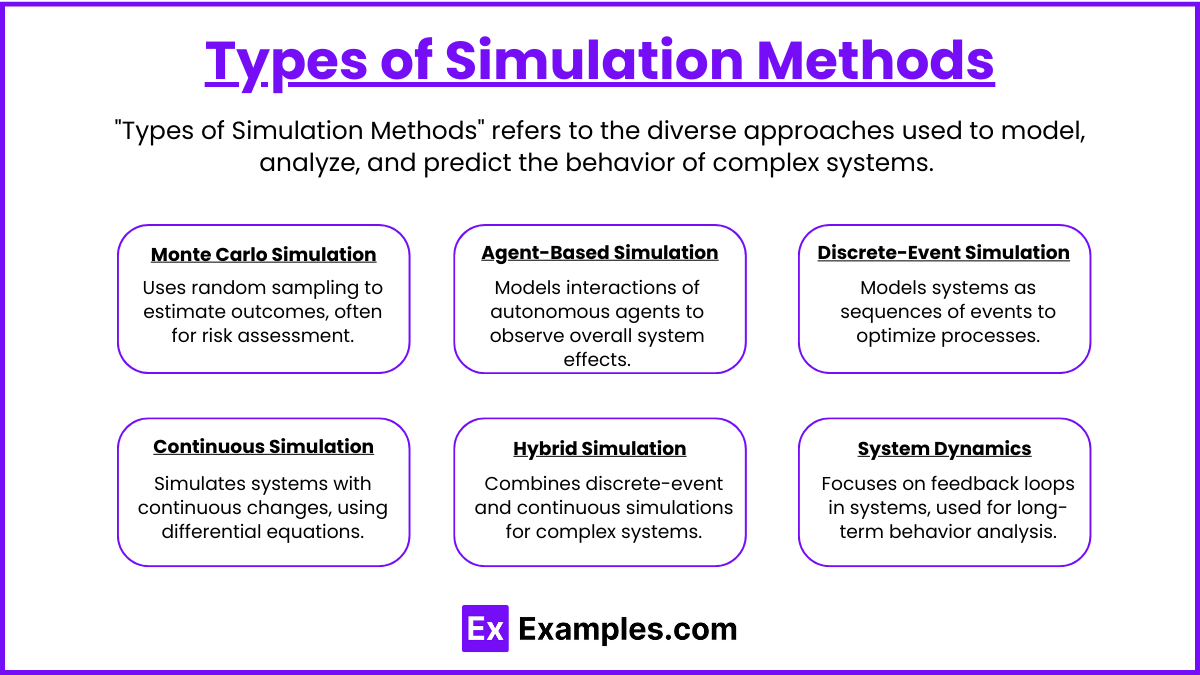
- Monte Carlo Simulation
Monte Carlo simulation involves using random sampling and statistical modeling to estimate mathematical functions and mimic the operation of complex systems. It’s widely used in finance, engineering, and risk analysis to assess the impact of uncertainty on decision-making. - Agent-Based Simulation
This type focuses on the interactions of autonomous agents (individuals, objects, or entities) within a defined environment. Agent-based models are commonly used to simulate social systems, biological processes, and economic behaviors, providing insights into how individual actions impact overall system behavior. - Discrete-Event Simulation
Discrete-event simulation models the operation of a system as a sequence of events in time. Each event occurs at a particular instant and marks a change in the system’s state. This method is frequently applied in logistics, manufacturing, and customer service operations to optimize workflows and identify bottlenecks. - Continuous Simulation
Continuous simulation models systems where changes occur continuously over time, often described by differential equations. It’s primarily used in fields like physics, biology, and environmental science to simulate systems like fluid dynamics, population growth, and chemical reactions. - Hybrid Simulation
Hybrid simulation combines discrete-event and continuous simulation methods to model complex systems that have both discrete and continuous characteristics. This approach is useful in industries where interactions between continuous processes and discrete events are critical, such as healthcare systems, energy production, and traffic flow analysis. - System Dynamics
System dynamics simulation focuses on the feedback loops and time delays that impact the behavior of complex systems over time. Used extensively in business, environmental studies, and public policy, system dynamics helps in understanding long-term behaviors like market fluctuations, resource management, and ecological sustainability.
Purposes of Simulation Methods
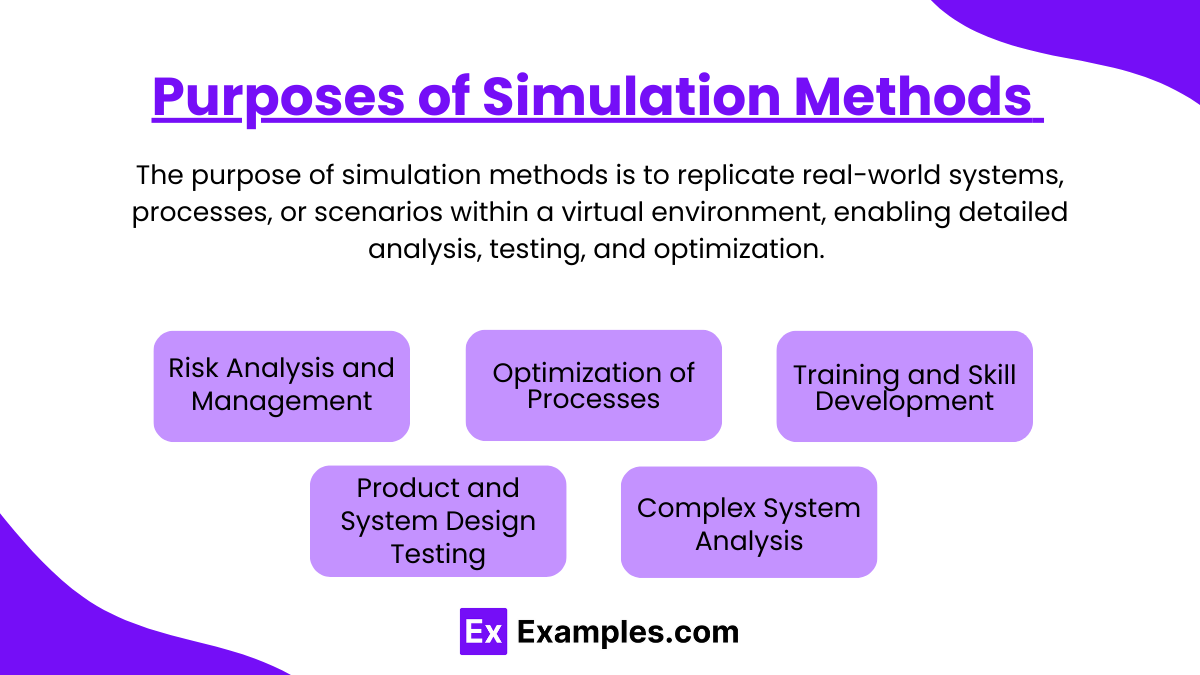
Simulation methods serve various purposes, particularly in contexts that require predictive analysis, decision-making under uncertainty, or system behavior assessment. Here are some key purposes of using simulation methods:
- Risk Analysis and Management
Simulation helps organizations identify, assess, and manage risks by modeling uncertain variables and forecasting potential outcomes, providing insights into scenarios that could impact decision-making and strategic planning. - Optimization of Processes
By simulating processes, businesses can identify inefficiencies and optimize operations without disrupting actual workflows, allowing for improved resource allocation and performance enhancement. - Training and Skill Development
Simulations create controlled, risk-free environments for training professionals, such as pilots or healthcare workers, allowing them to practice and refine their skills before applying them in real-life situations. - Product and System Design Testing
In engineering and product development, simulations allow for testing designs under various conditions, which helps reduce physical prototype costs and enhances reliability, quality, and safety before market release. - Complex System Analysis
Simulation methods model complex systems—like economies, ecosystems, or supply chains—enabling a deeper understanding of system behavior, interactions, and potential responses to changes.
Applications of Simulation Methods
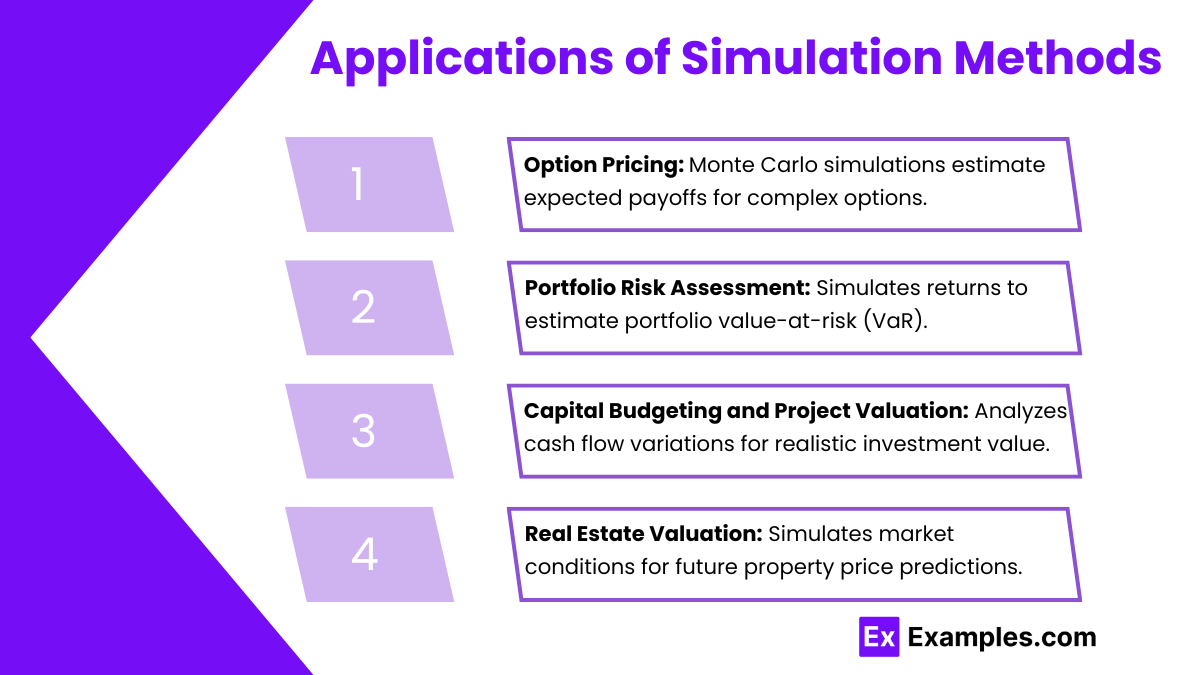
- Option Pricing: Monte Carlo simulations calculate the expected payoff of options, especially when dealing with complex derivatives where analytical solutions are impractical.
- Portfolio Risk Assessment: Helps in estimating value-at-risk (VaR) for portfolios by simulating returns and analyzing downside risk.
- Capital Budgeting and Project Valuation: Simulation helps to analyze potential cash flow variations in capital budgeting decisions, aiding in more realistic investment appraisals.
- Real Estate Valuation: Allows investors to simulate various market conditions and potential future property prices based on historical data.
Benefits of Using Simulation Methods
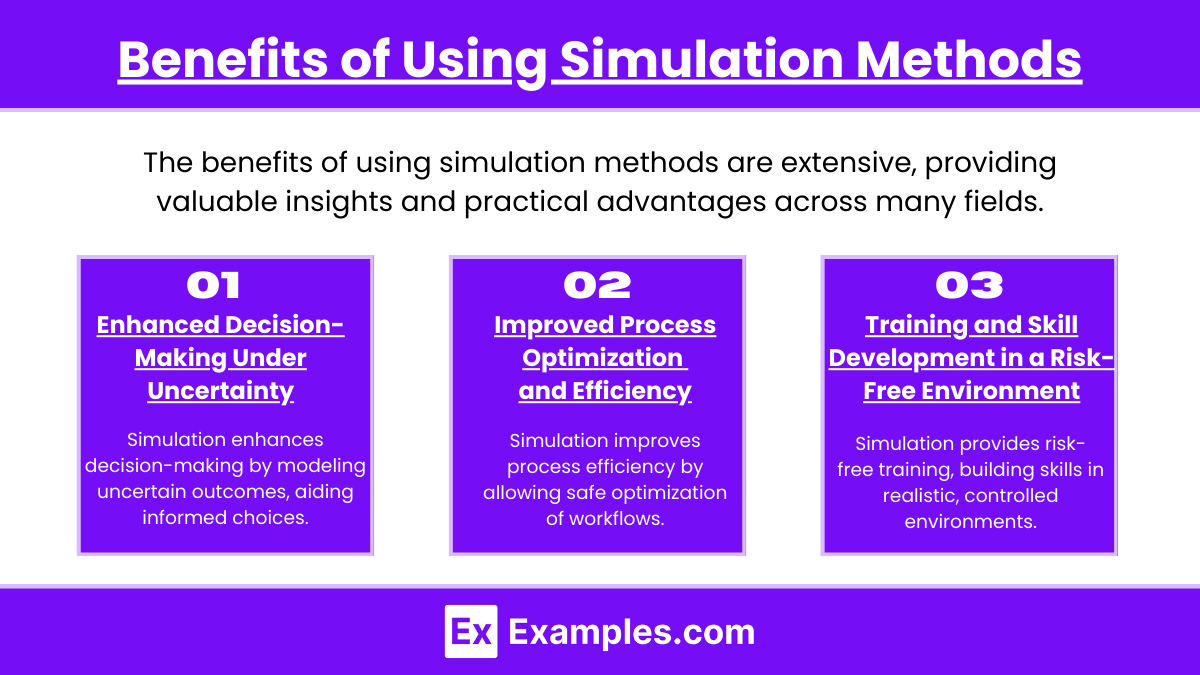
1. Enhanced Decision-Making Under Uncertainty
Simulation methods enable decision-makers to navigate uncertainties by modeling a range of potential outcomes and the probabilities associated with each. Unlike static models, simulations can account for various unpredictable variables, allowing businesses and organizations to test different scenarios before making costly decisions. For instance, in financial forecasting, simulations provide insight into potential market fluctuations, offering a more reliable foundation for investment strategies. This approach reduces the risk of unexpected losses and helps stakeholders make more informed decisions based on probable scenarios rather than relying on single-point forecasts or estimations.
2. Improved Process Optimization and Efficiency
In complex systems, such as manufacturing and logistics, simulation methods enable the testing and optimization of workflows without disrupting actual operations. By modeling these processes, organizations can identify bottlenecks, test new configurations, and assess resource allocation strategies without the costs or risks associated with live experimentation. This is especially valuable in high-stakes environments where errors are costly or safety is a concern. Simulation allows companies to refine their operations for optimal productivity, often leading to significant cost savings, enhanced safety, and improved output quality.
3. Training and Skill Development in a Risk-Free Environment
Simulation methods provide a controlled, risk-free environment for training and developing skills, especially in industries where real-world training could be dangerous or prohibitively expensive. For example, in healthcare, flight training, and military applications, simulations allow participants to engage in realistic scenarios where they can practice procedures, make decisions, and experience the consequences of those decisions without endangering lives or incurring high costs. This hands-on approach not only accelerates learning but also enhances the competence and confidence of trainees, making them better prepared for real-world situations.
Examples
Example 1. Financial Risk Management:
Simulation methods are widely employed in financial risk management, especially in assessing the potential risks associated with investment portfolios. By creating a range of possible financial scenarios, analysts can simulate the impact of different market conditions on a portfolio’s performance. Monte Carlo simulations, for instance, can help determine the likelihood of different returns, enabling better risk assessment and strategic planning.
Example 2. Healthcare and Epidemic Modeling:
In healthcare, simulation methods are critical for understanding the spread of diseases and evaluating intervention strategies. By simulating various epidemic scenarios, researchers can model the potential impact of public health measures like social distancing or vaccination programs. This enables policymakers to make informed decisions based on simulated outcomes of different healthcare strategies.
Example 3. Manufacturing Process Optimization:
In manufacturing, simulation methods help optimize production processes by testing different scenarios in a virtual environment. For instance, discrete event simulation can model the flow of materials and workforce dynamics, allowing companies to identify bottlenecks, streamline operations, and predict the impact of changes without disrupting the actual production line.
Example 4. Climate Change Impact Analysis:
Climate scientists use simulation methods to predict the long-term effects of climate change. By simulating global climate systems under different scenarios, scientists can estimate future temperature changes, sea level rise, and extreme weather events. These simulations inform environmental policies and help communities prepare for potential climate-related challenges.
Example 5. Urban Traffic Flow Modeling:
Simulation methods are extensively used in urban planning, particularly in traffic management and infrastructure development. By simulating traffic flow and vehicle behavior under various conditions, urban planners can predict congestion patterns and assess the effectiveness of infrastructure changes, such as new roads or public transport options, in reducing traffic congestion and improving urban mobility.
Practice Questions
Question 1
Which of the following best describes the purpose of Monte Carlo simulations in financial risk management?
A. To predict exact outcomes for specific investments
B. To analyze a single outcome for a given set of conditions
C. To assess potential risk by generating a range of possible outcomes
D. To create a deterministic model of financial markets
Answer: C. To assess potential risk by generating a range of possible outcomes
Explanation:
Monte Carlo simulations are used in financial risk management to estimate the range of potential outcomes for investments. This method is particularly useful in assessing risk because it involves running thousands of simulations with random variables to understand the likelihood of different financial scenarios. Unlike deterministic models, Monte Carlo simulations don’t predict exact outcomes; instead, they provide a probability distribution of possible results, helping analysts and investors make informed decisions about risk.
Question 2
In the context of healthcare, which type of simulation method is commonly used to model the spread of infectious diseases?
A. Agent-based modeling
B. Discrete event simulation
C. Monte Carlo simulation
D. Predictive analytics
Answer: A. Agent-based modeling
Explanation:
Agent-based modeling (ABM) is a popular simulation method for modeling the spread of infectious diseases. In ABM, individual “agents” (representing people or groups) are assigned specific behaviors and interactions within a simulated environment. This approach allows researchers to observe how infectious diseases may spread through populations based on individual actions, interactions, and varying health measures. Unlike Monte Carlo simulations, which are random but aggregate, agent-based models track each agent’s behavior, providing a detailed picture of disease dynamics and the potential effectiveness of interventions.
Question 3
What is the primary benefit of using simulation methods in manufacturing process optimization?
A. Minimizing the cost of physical experiments by testing scenarios virtually
B. Eliminating all errors in the production process
C. Guaranteeing that production targets are always met
D. Accurately predicting exact production output
Answer: A. Minimizing the cost of physical experiments by testing scenarios virtually
Explanation:
Simulation methods in manufacturing, such as discrete event simulation, allow companies to test and optimize production processes in a virtual environment. This approach helps identify bottlenecks, test various configurations, and make adjustments without the cost and disruption associated with physical trials. By simulating different scenarios, manufacturers can explore improvements and troubleshoot issues, ultimately enhancing efficiency and reducing costs. While simulation aids in optimizing the process, it doesn’t guarantee exact outcomes or eliminate all errors but provides valuable insights for more informed decision-making.