Preparing for the CMA requires a comprehensive understanding of Decision Analysis, essential for effective strategic planning and operational decision-making. Gaining proficiency in data analysis, quantitative methods, and decision-making techniques equips you with crucial skills for evaluating alternative courses of action and optimizing business outcomes. Key areas include cost-volume-profit analysis, risk management, linear programming, and the use of decision trees, all vital for achieving informed, data-driven decisions that enhance performance and drive organizational success, which is critical for excelling on the CMA exam and advancing in managerial roles.
Learning Objectives
In studying “Decision Analysis” for the CMA Exam, you should develop an understanding of the principles governing data interpretation, statistical tools, and decision-making frameworks. Explore the significance of quantitative techniques, sensitivity analysis, and scenario planning in driving informed business decisions. Evaluate how these elements contribute to assessing risks, identifying opportunities, and optimizing resource allocation. Additionally, grasp how these practices serve as a foundation for ethical decision-making and strategic leadership. Apply this knowledge to analyzing decision alternatives, forecasting outcomes, and solving CMA practice questions on break-even analysis, linear programming, and uncertainty management.
What is Decision Analysis?
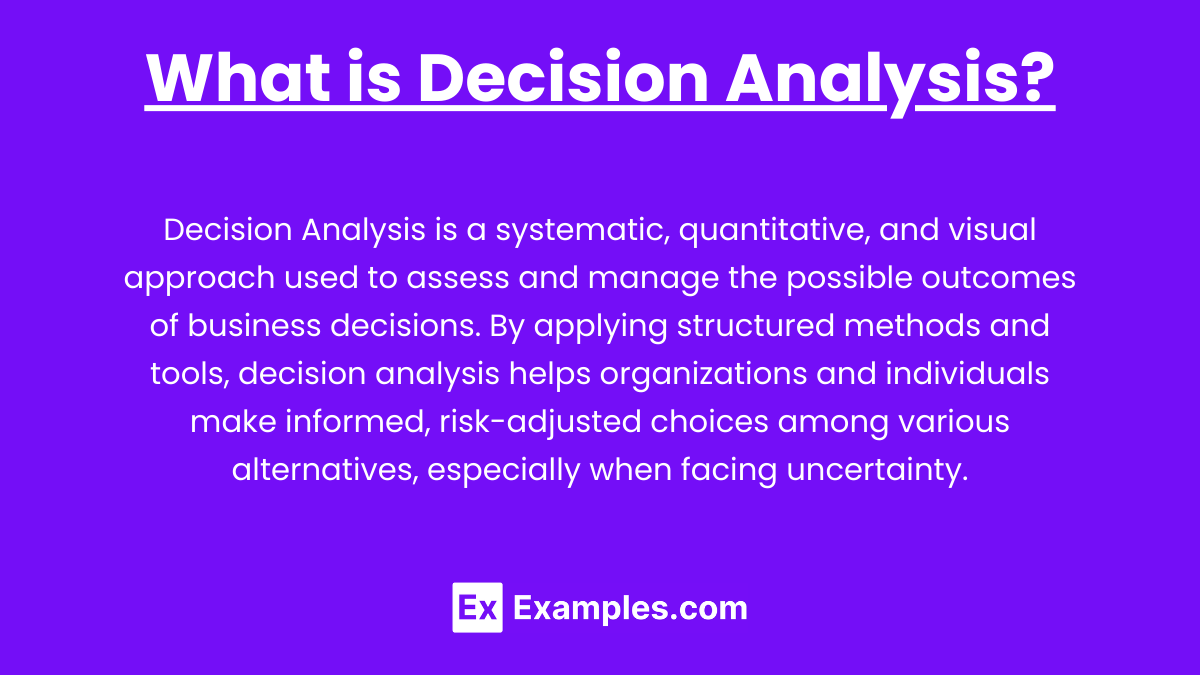
Decision Analysis is a systematic, quantitative, and visual approach used to assess and manage the possible outcomes of business decisions. By applying structured methods and tools, decision analysis helps organizations and individuals make informed, risk-adjusted choices among various alternatives, especially when facing uncertainty.
Purposes of Decision Analysis
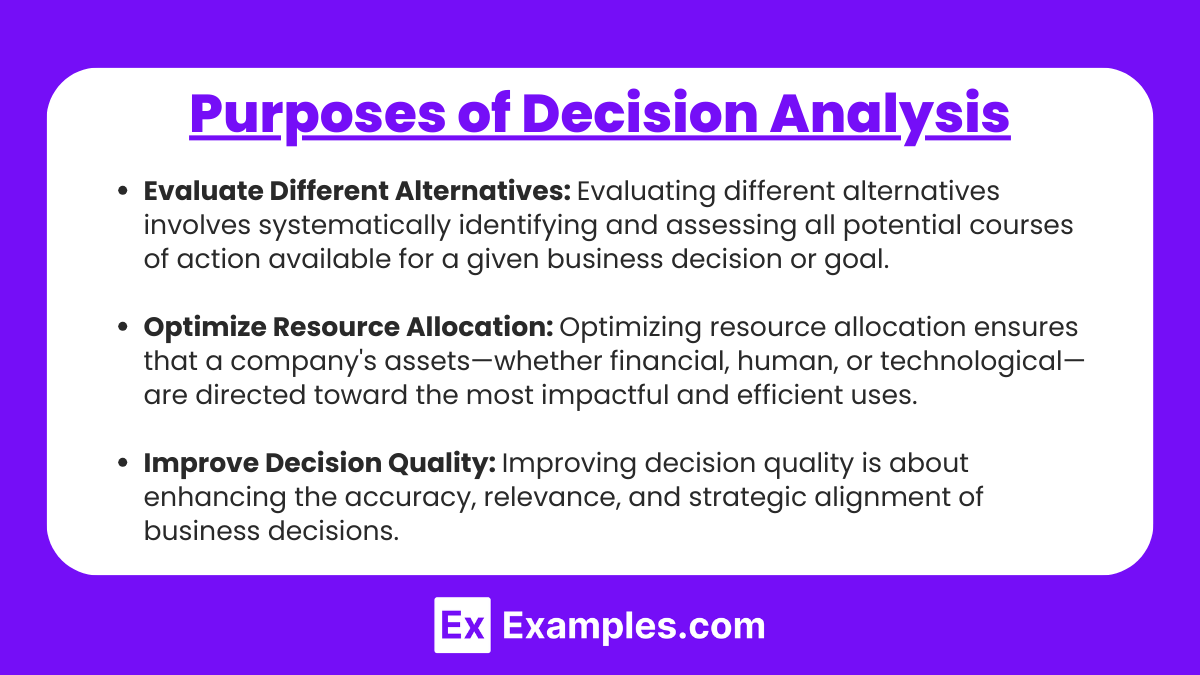
1. Evaluate Different Alternatives
Evaluating different alternatives involves systematically identifying and assessing all potential courses of action available for a given business decision or goal. This process begins with defining the decision criteria, which could include financial returns, alignment with company strategy, and impact on resources. By generating and evaluating a list of alternatives, decision-makers can weigh the pros and cons of each option, assessing feasibility, costs, and projected benefits. This approach allows businesses to make informed decisions that account for all possibilities, minimizing regret and maximizing the likelihood of choosing the most effective solution. Evaluating alternatives thoroughly supports strategic flexibility, enabling companies to adapt as conditions change or new information arises.
2. Optimize Resource Allocation
Optimizing resource allocation ensures that a company’s assets—whether financial, human, or technological—are directed toward the most impactful and efficient uses. This process involves prioritizing projects, initiatives, or departments based on their expected return on investment (ROI), alignment with strategic goals, and potential for growth. Effective resource allocation minimizes waste and maximizes value by focusing on high-impact activities and projects that drive the company forward. Businesses that optimize their resources can maintain operational efficiency, improve productivity, and enhance profitability. It also enables companies to better withstand economic fluctuations, making sure that resources are available when and where they’re needed most.
3. Improve Decision Quality
Improving decision quality is about enhancing the accuracy, relevance, and strategic alignment of business decisions. By incorporating data analysis, risk assessment, and market insights, organizations can make well-informed choices that better reflect the realities of their industry and operational environment. This process also involves reducing biases and ensuring decisions are aligned with long-term objectives. Improved decision quality often requires investing in tools, training, and processes that enhance analytical capabilities, increase access to reliable data, and facilitate collaboration. High-quality decisions drive better business outcomes by reducing errors, increasing alignment across departments, and fostering a proactive, data-driven culture.
4. Minimize Risks
Minimizing risks entails identifying, analyzing, and mitigating uncertainties that could adversely affect business outcomes. This process includes risk assessment techniques to determine the likelihood and impact of potential threats, as well as the implementation of risk management strategies such as diversification, insurance, or contingency planning. By proactively managing risks, companies can reduce the likelihood of negative financial, operational, or reputational impacts. Minimizing risks also enables organizations to take calculated risks more confidently, knowing that they have measures in place to protect against significant downsides. Effective risk management creates resilience and stability, allowing businesses to navigate uncertainties with greater confidence and flexibility.
5. Increase Profitability
Increasing profitability focuses on strategies to maximize financial performance by enhancing revenue streams, reducing costs, and optimizing operational efficiency. This goal involves identifying and capitalizing on high-margin opportunities, improving productivity, and streamlining processes. Strategies to increase profitability include expanding into new markets, enhancing product or service offerings, and implementing cost-saving initiatives. A focus on profitability ensures that the company can not only meet its financial obligations but also reinvest in growth, innovation, and talent development. Sustainable profitability is crucial for long-term success, as it provides the financial foundation for continued expansion, increased shareholder value, and the ability to adapt in competitive markets.
Types of Decision Analysis
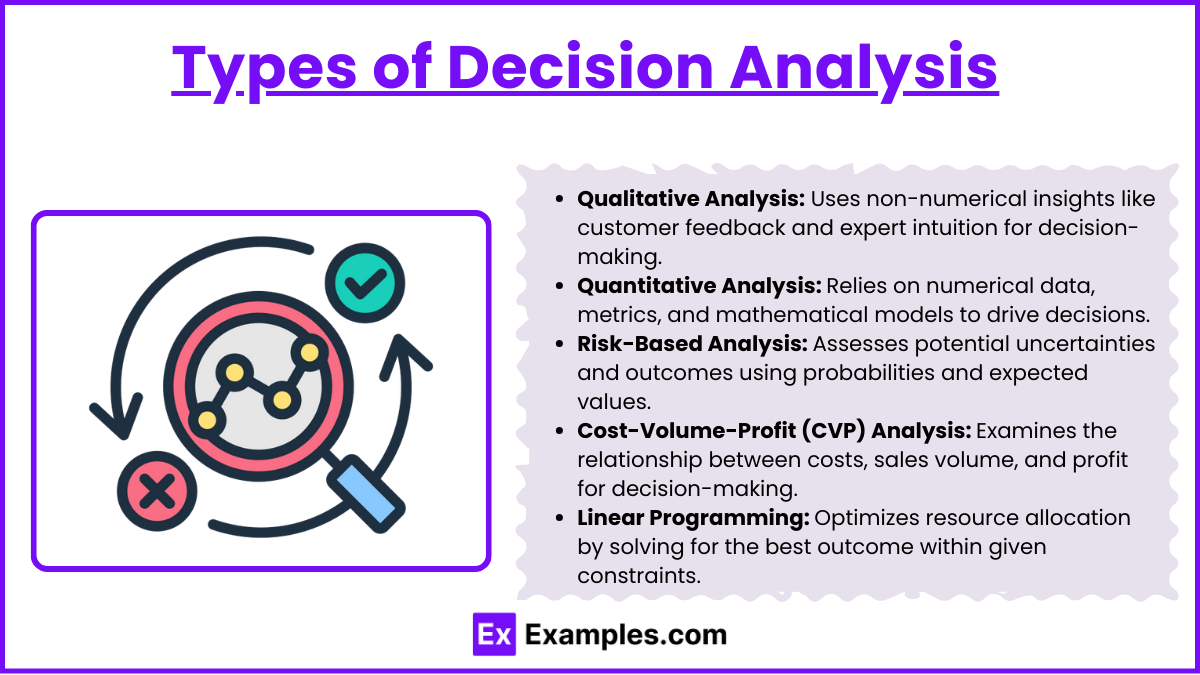
1. Qualitative Analysis
Qualitative analysis uses non-quantifiable information, such as customer feedback, employee insights, and management intuition, to guide decision-making. This approach focuses on understanding context, motivations, behaviors, and sentiments that can’t be easily measured. For example, customer reviews and focus group discussions can provide insights into how a product is perceived and suggest potential improvements. Similarly, management experience and industry knowledge can offer valuable perspectives that may not be reflected in numbers alone. Qualitative analysis is especially useful in scenarios where human factors, brand perception, and market trends play a significant role. By incorporating this analysis, businesses gain a well-rounded understanding of situations, complementing the data-driven insights from quantitative methods.
2. Quantitative Analysis
Quantitative analysis involves the use of numerical data, mathematical models, and statistical techniques to make decisions and evaluate options. This analysis typically includes metrics such as the payback period, net present value (NPV), internal rate of return (IRR), and break-even analysis. Quantitative methods provide objective insights, allowing businesses to measure and forecast outcomes based on hard data. For instance, NPV is often used to assess investment opportunities by calculating the value of future cash flows in today’s terms. Quantitative analysis is essential for cost-benefit assessments, financial modeling, and performance measurement, as it enables companies to make data-driven decisions and minimize biases. By relying on metrics and calculations, quantitative analysis helps businesses maximize returns and allocate resources effectively.
3. Risk-Based Analysis
Risk-based analysis evaluates uncertainties associated with potential decisions by assessing probabilities, outcomes, and their expected values. This approach is especially valuable in decision-making scenarios where potential risks and rewards are significant. Techniques like Monte Carlo simulations, sensitivity analysis, and risk matrices are commonly used to model uncertainties and analyze different scenarios. By quantifying risks, businesses can better understand the likelihood and impact of various outcomes and choose options that align with their risk tolerance. Risk-based analysis enables companies to identify and manage potential downsides proactively, increasing resilience and ensuring more reliable, informed decision-making even in volatile conditions.
4. Cost-Volume-Profit (CVP) Analysis
Cost-Volume-Profit (CVP) analysis examines the relationship between costs, sales volume, and profit to help businesses make informed decisions about pricing, production, and sales strategies. CVP analysis determines the break-even point, or the sales level at which total revenue equals total costs, resulting in zero profit or loss. It also assesses how changes in selling price, fixed costs, variable costs, and sales volume impact profitability. CVP is particularly useful for setting pricing strategies, deciding on product mix, and evaluating the financial impact of changes in production levels. By understanding the dynamics between cost, volume, and profit, companies can make better decisions that optimize profitability and improve financial stability.
5. Linear Programming
Linear programming is a mathematical optimization technique used to allocate resources efficiently while satisfying certain constraints. This approach involves defining an objective function—typically maximizing profit or minimizing costs—and identifying constraints related to resources, such as labor, materials, budget, or time. By setting up and solving linear equations, businesses can determine the optimal way to allocate limited resources to achieve the desired outcome. Linear programming is widely used in operations, production planning, logistics, and supply chain management. For example, it can help a manufacturing company decide the ideal mix of products to produce within capacity limits to maximize profits. Through linear programming, organizations achieve resource optimization, streamline operations, and enhance decision-making accuracy in complex scenarios.
Examples
Example 1. Strategic Planning in a Manufacturing Company
In a manufacturing company, decision analysis is utilized to evaluate different strategies for increasing production efficiency. By analyzing data on current production costs, resource allocation, and market demand, decision-makers can identify optimal approaches, such as whether to invest in new machinery, streamline processes, or reallocate staff. The analysis involves comparing potential outcomes, costs, and risks associated with each option, helping management choose the strategy that maximizes profitability while minimizing operational risks.
Example 2. Investment Portfolio Optimization
Financial institutions often use decision analysis to guide investment portfolio decisions. By evaluating various factors like expected returns, risk levels, and market trends, decision analysis helps investment managers select a balanced portfolio. It involves comparing different asset classes, such as stocks, bonds, or real estate, and determining the mix that aligns with the investor’s risk tolerance and financial goals. The analysis supports making informed decisions to achieve optimal returns while managing investment risks effectively.
Example 3. Product Launch in Tech Industry
A tech company can apply decision analysis when launching a new product. The process involves evaluating different launch strategies based on potential market reception, costs, and competition. By analyzing customer surveys, competitor performance, and cost projections, the company can decide whether to launch the product regionally, nationally, or globally. Decision analysis ensures that the launch strategy aligns with the company’s market objectives, budget constraints, and long-term growth plans, increasing the likelihood of a successful product launch.
Example 4. Healthcare Treatment Options
In the healthcare sector, decision analysis is used to evaluate treatment options for patients. For instance, doctors might analyze different medical interventions for a patient based on factors such as effectiveness, potential side effects, costs, and patient preferences. By using a decision tree model, healthcare providers can visualize outcomes and select the treatment that offers the best balance of efficacy and patient quality of life. Decision analysis supports patient-centered care by helping medical professionals make evidence-based treatment choices.
Example 5. Supply Chain Management in Retail:
Retailers use decision analysis to optimize their supply chains. By analyzing data related to supplier performance, inventory levels, logistics costs, and demand forecasts, decision-makers can decide how to improve supply chain efficiency. The analysis might reveal whether to increase stock levels of popular products, find alternative suppliers, or adjust distribution methods. This approach ensures that retail companies maintain optimal inventory levels, minimize costs, and respond effectively to changing consumer demands.
Practice Questions
Question 1
In decision analysis, the concept of “risk aversion” implies that:
A. The decision-maker is willing to take on high-risk projects for high returns
B. The decision-maker prefers certain outcomes over uncertain ones, even with potentially lower returns
C. The decision-maker has no preference between risk and return
D. The decision-maker relies solely on probability without considering outcomes
Answer: B. The decision-maker prefers certain outcomes over uncertain ones, even with potentially lower returns
Explanation:
Risk aversion refers to the tendency of decision-makers to choose options with lower risk when faced with uncertain outcomes, even if the certain option offers lower returns. A risk-averse individual values predictability and is willing to sacrifice potential higher gains for a more secure, known outcome. This behavior contrasts with risk-seeking, where a decision-maker might prefer higher-risk options for potentially greater returns. Risk aversion is a crucial consideration in decision analysis, as it shapes decisions involving uncertainty.
Question 2
A decision tree is often used in decision analysis to:
A. Calculate probabilities only
B. Illustrate sequential decisions and possible outcomes
C. Identify the minimum-cost option
D. Focus solely on qualitative data
Answer: B. Illustrate sequential decisions and possible outcomes
Explanation:
A decision tree visually represents decisions and possible outcomes in a sequence, which helps decision-makers evaluate the financial impact of different paths. Each branch represents a decision or event with associated probabilities and payoffs, allowing for an organized approach to analyze potential outcomes and identify optimal decisions. Decision trees are especially useful in scenarios with uncertainty and multiple decision points.
Question 3
When performing a sensitivity analysis in decision analysis, what is the primary purpose?
A. To predict the exact outcome of a decision
B. To assess how different values of a variable impact the outcome
C. To determine the best-case scenario outcome only
D. To increase the decision-maker’s bias towards a preferred outcome
Answer: B. To assess how different values of a variable impact the outcome
Explanation:
Sensitivity analysis evaluates how the changes in key variables (such as costs, probabilities, or payoffs) affect the overall outcome of a decision. This is critical in decision analysis, as it highlights which variables have the most influence on results and helps decision-makers understand the robustness of their choice under varying conditions. This method does not predict exact outcomes but provides insight into the reliability of the decision given potential fluctuations.